AI Engineering Bootcamp for Programmers
আপনি যদি পাইথনের বেসিক জানেন, তাহলে এবার সময় এসেছে সেই স্কিলকে লেভেল আপ করার—AI Engineering Bootcamp for Programmers কোর্সের মাধ্যমে। পাবেন একদম লাইভে হাতে-কলমে শেখার এক্সপেরিয়েন্স যেখানে আপনি শিখবেন AI ইঞ্জিনিয়ার হওয়ার জন্য প্রয়োজনীয় সবকিছু—Python প্রোগ্রামিং, ম্যাথমেটিক্স, স্ট্যাটিস্টিক্স, NLP, NLU, Computer Vision (CV), LLM, Langchain, Vector Database, Explainable AI (XAI)—একটাও বাদ যাবে না। কোর্স শেষে আপনার পোর্টফোলিওতে থাকবে ১২ টি প্রোজেক্ট। AI Engineer হিসেবে মার্কেটে এন্ট্রি নেবার জন্য আপনার পোর্টফোলিওকে করবে ইন্ডাস্ট্রি রেডি। ব্লকব্লাস্টার AI ইঞ্জিনিয়ার হিসেবে নিজেকে গড়ে তোলার টাইম এটাই- AI এর এই ট্রেইন মিস করা যাবে না কিন্তু।

ক্লিক করে দেখে নিন কোর্সের ডেমো ক্লাস
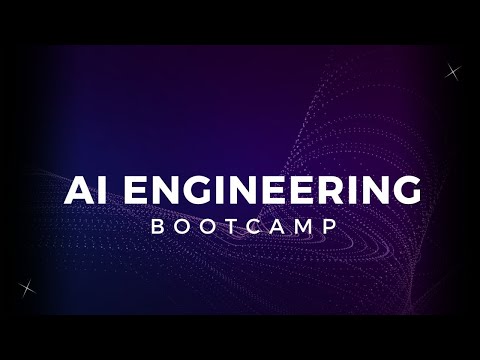
৪ দিন বাকি
৫৫ সিট বাকি
এই কোর্সে আপনি পাচ্ছেন
৫ মাসের স্টাডি প্ল্যান
৪০ টি লাইভ ক্লাস
১২ টি রিয়াল লাইফ প্রোজেক্ট
পাইথন প্রোগ্রামিং
ম্যাথমেটিক্স, স্ট্যাটিসটিক্স, ক্যালকুলাস
Natural Language Processing
Large Language Model
Langchain
Vector Database
Speech Recognition
উইকলি অ্যাসেসমেন্ট
লাইফটাইম এক্সেস
সার্টিফিকেট
জব প্লেসমেন্ট সাপোর্ট
কল করুন +88013038047939
(সকাল ১০টা থেকে রাত ১০টা)
ব্যাচ ১
শুরু হবে
শনি, ১০ মে
ক্লাস শিডিউল
শনি,
মঙ্গল,
(রাত ৯:০০ - ১০:৩০)
ব্যাচ ১
ক্লাস শিডিউল
শনি,
মঙ্গল,
(রাত ৯:০০ - ১০:৩০)
ফ্রি ডেমো ক্লাস
AI Engineering Job Roadmap: Skills, Projects & CV that Get You Hired
৬ মে
রাত ৯:৩০টা
স্টাডি প্ল্যান
১৯ টি মডিউল
৪০ টি লাইভ ক্লাস
Programming, Math, and Tools Foundation (Module 0-3)
ক্লাস নিবেনঃ
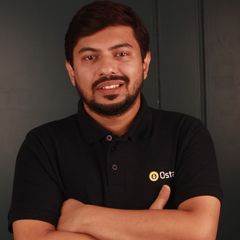
Jaid Jashim
সপ্তাহ
০
শুরুর আগে শুরু
59 recorded video
ব্যাচের কার্যক্রম - মেইন লাইভ ক্লাস শুরুর আগেই যারা ইনরোল করেছেন, তারা কি বসে থাকবেন ? না! এই মডিউলে ব্যাসিক বেশ কিছু ভিডিও দেওয়া আছে। শুরু হয়ে যাক তবে শেখার জার্নি!
সপ্তাহ
১
APIs, File Handling, and Data Manipulation

2 live class

1 Quiz
Objective: Enable students to collect and manipulate real-world data.
Live Class 1: What is a CSV file | Reading CSV using csv.reader() | Reading rows as dictionary using csv.DictReader() | What is JSON | Reading JSON file using json.load() | Converting JSON string using json.loads() | File modes: r, w, a | Reading text file using open() | Writing text file using write() | Using with statement for safe file handling
Live Class 2: API Requests with requests module | Making GET and POST requests | Handling API response data | Basics of web scraping | Using requests and BeautifulSoup | Extracting data from HTML elements | Introduction to data cleaning | Handling missing values with Pandas | Removing duplicates and irrelevant columns | Formatting and transforming data types
সপ্তাহ
২
Math for Machine Learning

2 live class

1 Quiz
Objective: Build math foundations for machine learning models.
Live Class 1: Introduction to vectors | Vector addition and scalar multiplication | Understanding matrices | Matrix dimensions and structure | Matrix operations: addition and multiplication | Dot product between vectors | Geometric interpretation of dot product | Applications of dot product in data science
Live Class 2: Introduction to probability concepts | Basic rules of probability | What is statistics and its role in data analysis | Calculating mean and median | Understanding variance and standard deviation | Introduction to data distributions | Normal distribution and its properties | Other common distributions: binomial, Poisson
সপ্তাহ
৩
Development Tools and Best Practices

2 live class

1 Quiz
Objective: Introduce Git, Jupyter, Colab, and environment setup.
Live Class 1: What is Git and why use it | Basic Git commands: init, add, commit | Understanding version control | What is GitHub and how it works | Creating and managing repositories on GitHub | Cloning and pushing code | Branching and merging basics | Typical project folder structure | Best practices for organizing codebase
Live Class 2: What is Jupyter Notebook | Running and managing code cells | Introduction to Google Colab | Uploading and sharing notebooks | Writing clean and readable code | Using comments and proper indentation | Writing Markdown in notebooks | Formatting text, lists, and code blocks with Markdown | Creating headings and notes for better understanding
Machine Learning and Deep Learning Core (Module 4-10)
ক্লাস নিবেনঃ
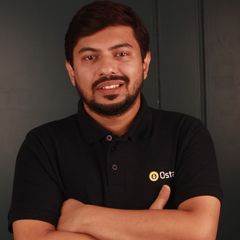
Jaid Jashim
সপ্তাহ
৪
Regression Models and Evaluation

2 live class

1 Quiz
Live Class 1: Introduction to linear regression | Fitting a line to data using least squares | Understanding slope and intercept | What is overfitting in regression | Introduction to regularization | Ridge regression and L2 penalty | Lasso regression and L1 penalty | Comparing ridge and lasso | When to use ridge vs lasso
Live Class 2: Introduction to model evaluation | What is Mean Absolute Error (MAE) | Interpreting MAE in regression models | What is Root Mean Squared Error (RMSE) | Difference between MAE and RMSE | What is R-squared (R²) | Interpreting R² as model accuracy | Choosing the right metric for evaluation
Project: House Price Predictor
সপ্তাহ
৫
Classification Models and Evaluation

2 live class

1 Quiz
Live Class 1: Introduction to logistic regression | Understanding sigmoid function and probabilities | Binary classification with logistic regression | Introduction to K-Nearest Neighbors (KNN) | How KNN makes predictions based on distance | Choosing the right value of K | Introduction to decision trees | Splitting criteria: Gini and Entropy | Overfitting and pruning in decision trees | Comparing logistic, KNN, and decision trees
Live Class 2: What is a confusion matrix | Understanding TP, FP, TN, FN | Calculating precision and recall | What is F1 score and why it matters | Balancing precision and recall | Introduction to ROC curve | Interpreting ROC-AUC score | Choosing metrics based on problem type
Project: Diabetes Detection System
সপ্তাহ
৬
Unsupervised Learning and Dimensionality Reduction

2 live class

1 Quiz
Live Class 1: Introduction to K-Means clustering | How K-Means groups similar data points | Choosing number of clusters | What is the Elbow Method | Using Elbow Method to find optimal K | Introduction to PCA (Principal Component Analysis) | Reducing dimensionality with PCA | Interpreting principal components | PCA vs clustering: when to use what
Live Class 2: Introduction to customer segmentation | Importance of segmenting customers | Clustering customers based on behavior or demographics | Using K-Means for customer segmentation | Visualizing clusters with scatter plots | Interpreting the segmented data | Visualizing customer profiles using bar charts and histograms | Using heatmaps for correlation analysis
Project: Customer Segmentation Engine
সপ্তাহ
৭
Introduction to Deep Learning

2 live class

1 Quiz
Live Class 1: Introduction to neural networks | Structure of a neural network: neurons, layers, activation functions | Forward propagation process | Calculating outputs in a neural network | What is backpropagation | Adjusting weights during backpropagation | Gradient descent and learning rate | Role of loss function in training | Training a neural network through epochs
Live Class 2: Introduction to activation functions | Common activation functions: Sigmoid, ReLU, Tanh | Why activation functions are needed in neural networks | Introduction to loss functions | Mean Squared Error and Cross-Entropy Loss | How loss functions guide model training | Implementing a simple neural network | Forward propagation in a simple NN | Using backpropagation to update weights | Training the model with gradient descent
Project: MNIST Digit Classifier
সপ্তাহ
৮
Computer Vision with CNNs

2 live class

1 Quiz
Live Class 1: Introduction to Convolutional Neural Networks (CNN) | What is computer vision? Use cases in industry | Basics of CNNs: convolution, pooling, flatten, FC | CNN architecture overview: input, convolution, pooling, fully connected layers | What is a convolution layer and its purpose | Applying filters to input images | Understanding kernel size and stride | Max pooling and average pooling layers | Reducing spatial dimensions with pooling | Role of convolution and pooling in feature extraction
Live Class 2: Introduction to image classification | Overview of CNN for image classification | Using Keras for building CNN models | Loading and preprocessing image data | Defining the CNN architecture in Keras | Adding convolution, activation, and pooling layers | Flattening and adding fully connected layers | Compiling the model with optimizer, loss, and metrics | Training the model with image data | Evaluating model performance on test data | Image preprocessing techniques: resizing, normalization | Data augmentation for Image Data
Project: Dog vs Cat Image Classifier
সপ্তাহ
৯
Recurrent Neural Networks and Time-Series Forecasting

2 live class

1 Quiz
Live Class 1: Introduction to Recurrent Neural Networks (RNN) | How RNNs handle sequential data | Vanishing gradient problem in RNNs | Introduction to Long Short-Term Memory (LSTM) networks | How LSTMs address the vanishing gradient issue | Structure of LSTM cells: forget, input, and output gates | Using RNNs and LSTMs for time series and text data | Sequential data processing with RNNs and LSTMs | Applications of RNN and LSTM in NLP and time series analysis
Live Class 2: Introduction to time-series forecasting | Using LSTM for sequential data prediction | Preprocessing time-series data for LSTM | Reshaping data for LSTM input | Defining LSTM architecture for forecasting | Training the LSTM model on time-series data | Evaluating the model with loss functions | Making predictions using the trained LSTM model | Visualizing forecast results vs actual values | Fine-tuning the model for better performance
Project: Sales Prediction Tool
সপ্তাহ
১০
Model Deployment & Monitoring with FastAPI, Docker and MLflo

2 live class

1 Quiz
Live Class 1: Building Scalable ML APIs with FastAPI
What is FastAPI and why it's ideal for ML deployment | Setting up a FastAPI app for serving predictions | Creating robust endpoints with Pydantic for validation | Serving ML models using joblib or pickle | Structuring APIs: /predict, /health, /info endpoints | Testing APIs using Swagger UI, ReDoc, and Postman | Intro to async handling and real-time performance advantages
Live Class 2: Dockerizing Your ML Service & Intro to MLflow
Docker Focus: | What is Docker and why use it for ML APIs | Writing a Dockerfile for FastAPI apps | Building Docker images and running containers | Exposing containerized APIs over localhost/port | Testing containerized predictions with curl/Postman | Analyzing API responses and troubleshooting | Basics of cloud deployment | Deploying Dockerized applications on cloud platforms | Using services like AWS, Heroku, or Google Cloud for deployment
Project: Production Ready ML Model Pipeline with FastAPI, Docker & MLflow
NLP, Transformers, LLMs, and Tool-Integrated Applications (Module 11-17)
ক্লাস নিবেনঃ
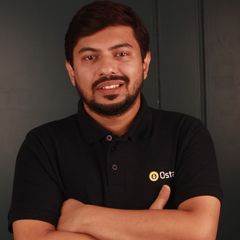
Jaid Jashim
সপ্তাহ
১১
Natural Language Processing Fundamentals

2 live class

1 Quiz
Live Class 1: Introduction to text preprocessing | What is tokenization and how it works | Breaking text into words or sentences | Introduction to lemmatization | Reducing words to their base form using lemmatization | What are stopwords and why remove them | Identifying and filtering stopwords in text | Using libraries like NLTK or SpaCy for preprocessing | Combining tokenization, lemmatization, and stopwords removal for clean text data
Live Class 2: Introduction to word embeddings | What is TF-IDF (Term Frequency-Inverse Document Frequency) | How TF-IDF captures word importance in a corpus | Introduction to Word2Vec and its two models: Continuous Bag of Words (CBOW) and Skip-gram | Training Word2Vec on text data to create word embeddings | What is GloVe (Global Vectors for Word Representation) | How GloVe differs from Word2Vec and captures global word relationships | Using pre-trained embeddings for NLP tasks | Comparing TF-IDF, Word2Vec, and GloVe for various applications
Project: Sentiment Analysis on Product Reviews
সপ্তাহ
১২
Transformers and Hugging Face Models

2 live class

1 Quiz
Live Class 1: Introduction to the attention mechanism | How attention allows models to focus on important parts of the input | Types of attention: hard and soft attention | Self-attention and its role in sequence processing | Introduction to Transformer architecture | Key components of Transformers: Encoder and Decoder | Multi-head attention mechanism | Positional encoding to preserve sequence order | Advantages of Transformers over RNNs and LSTMs | Applications of Transformers in NLP tasks like translation and summarization
Live Class 2: Introduction to Hugging Face Transformers | Overview of BERT and GPT models | Understanding pre-trained models and transfer learning | Fine-tuning BERT for text classification tasks | Preparing the dataset for fine-tuning | Training BERT with custom data using Hugging Face’s Trainer API | Fine-tuning GPT for text generation tasks | Adjusting hyperparameters during fine-tuning | Evaluating model performance on downstream tasks | Using pre-trained Hugging Face models for various NLP applications
Project: Text Classification Using Transformers
সপ্তাহ
১৩
Prompt Engineering and GPT APIs

2 live class

1 Quiz
Live Class 1: Introduction to prompt patterns in NLP | Designing effective prompts for different tasks | Few-shot learning and how it reduces data requirements | Understanding few-shot, one-shot, and zero-shot learning | Introduction to OpenAI API | Overview of GPT models and their capabilities | Making API requests to OpenAI for text generation and understanding tasks | Prompt engineering for better results | Fine-tuning models with custom prompts for specific tasks | Using OpenAI API in real-world applications like chatbots, summarization, and translation
Live Class 2: Introduction to function calling with GPT models | Using GPT for structured outputs like JSON, tables, or lists | Designing prompts for structured responses | Calling external functions or APIs from within a prompt | Handling structured data in response formats | Parsing and formatting structured outputs from GPT | Integrating GPT with external systems for dynamic function calls | Use cases of function calling in automation, data extraction, and decision-making tasks | Best practices for optimizing structured output quality
Project: Resume Generator Using GPT
সপ্তাহ
১৪
Introduction to LangChain

2 live class

1 Quiz
Live Class 1: Introduction to chains in NLP workflows | Combining multiple tasks or steps using chains | Using memory in AI models for context retention | Implementing memory to maintain state across interactions | Creating prompt templates for consistent input formatting | Benefits of reusable prompt templates in large-scale applications | How chains and memory work together in task automation | Example use cases of chains, memory, and templates in complex tasks like conversation bots and multi-step reasoning | Optimizing performance with chains and templates
Live Class 2: Introduction to CSV/PDF Q&A pipelines | Extracting data from CSV files for Q&A tasks | Parsing PDF files for text-based question answering | Using OCR for extracting text from scanned PDFs | Building a pipeline to answer questions from structured or unstructured documents | Document indexing for fast retrieval | How to index documents for efficient search and retrieval
Project: Chatbot for Document-Based QA
সপ্তাহ
১৫
Building Intelligent Tool-Integrated Applications

2 live class

1 Quiz
Live Class 1: Introduction to LangChain Agent Executor | How the Agent Executor manages complex tasks | Using LangChain to build intelligent agents | Creating and integrating custom tools into LangChain | Leveraging external APIs and services as custom tools | Implementing intermediate memory for maintaining context across tasks | Storing temporary information to guide agent decision-making | Optimizing agent performance with efficient memory management | Example use cases of LangChain in conversational agents and workflow automation
Live Class 2: Introduction to API tools in LangChain | Using built-in API tools for integrating external services | Handling API requests and responses with LangChain | Introduction to file tools for managing document-based inputs | Reading, writing, and processing files in pipelines | Using LangChain to process and analyze file data (CSV, JSON, text) | Error handling strategies in LangChain workflows | Catching and managing exceptions in API and file tool interactions | Debugging and logging in LangChain | Best practices for building robust workflows with error handling
Project: Business Assistant Chatbot
সপ্তাহ
১৬
Multi-Agent Systems and Workflow Automation

2 live class

1 Quiz
Live Class 1: Introduction to CrewAI | How CrewAI enables automation and collaboration across tasks | Overview of AutoGen and its role in automating task generation | Using AutoGen for dynamic task creation and assignment | Task delegation strategies for optimizing workflow | Assigning tasks based on skills, priorities, and timelines | Managing task dependencies and ensuring smooth handoffs | Best practices for delegating tasks effectively within automated systems
Live Class 2: Introduction to multi-agent systems | How multiple agents interact and collaborate to solve problems | Example 1: Multi-agent system for recommendation engines | Agents working together to provide personalized suggestions | Example 2: Collaborative robots in manufacturing | Coordinating tasks between robots to improve efficiency | Example 3: Autonomous vehicle fleet management | Multiple agents managing traffic flow and navigation
Project: Research Planning and Summarization Assistant
সপ্তাহ
১৭
Retrieval-Augmented Generation and Vector Databases

2 live class

1 Quiz
Live Class 1: Introduction to FAISS (Facebook AI Similarity Search) | Using FAISS for efficient similarity search and nearest neighbor search | How FAISS works with high-dimensional data | Introduction to Chroma for scalable vector storage | Using Chroma for real-time, fast retrieval of vector-based data | Overview of LangChain retriever | Integrating LangChain with FAISS and Chroma for document retrieval | How LangChain handles retrieval-augmented generation (RAG) tasks | Benefits of combining FAISS, Chroma, and LangChain for high-performance information retrieval | Use cases in document search, semantic search, and knowledge extraction
Live Class 2: Introduction to the full Retrieval-Augmented Generation (RAG) pipeline | Ingestion of data for RAG systems: collecting and processing raw data | Preprocessing data for embedding generation | Using embeddings to represent data in vector space | Introduction to embeddings: how they capture semantic meaning | Generating embeddings with models like BERT or Sentence Transformers | Storing and indexing embeddings for efficient retrieval | Retrieval process: searching for relevant documents or data based on query
Project: Knowledge Base Chatbot with Vector DB
ক্লিক করে দেখে নিন কোর্সের ডেমো ক্লাস

ক্লিক করে দেখে নিন কোর্সের ডেমো ক্লাস
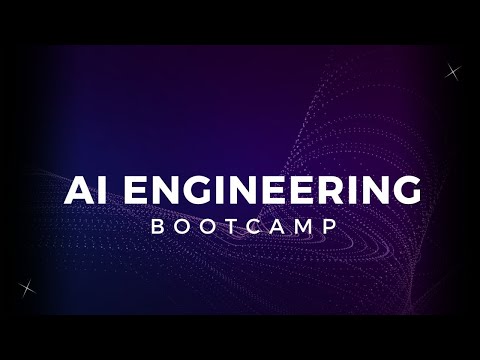
ইন্সট্রাক্টর

লিড ইন্সট্রাক্টর
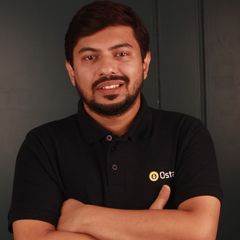
Jaid Jashim
Senior AI Engineer at InfiniBit | Senior Software Engineer – AI & ML at Dexian Limited, Bangladesh | Machine Learning Engineer at REVE Systems
কোর্স সম্পর্কে
যদি পাইথন প্রোগ্রামিং এর উপর ব্যাসিক নলেজ আপনার থাকে, তাহলে AI Engineering Bootcamp for Programmers লাইভ কোর্সটি আপনার জন্যই।
এই কোর্সটা কাদের জন্য?
যদি আপনি Python প্রোগ্রামিংয়ের বেসিকটা জানেন, আর ভাবছেন – “AI নিয়ে কিছু শিখলে ভালো হতো!”
আপনি যদি বিশ্ববিদ্যালয়ের স্টুডেন্ট হন, কিংবা ফ্রেশ গ্র্যাজুয়েট, অথবা এমন কেউ যিনি প্রোগ্রামিং জানেন কিন্তু AI নিয়ে কাজ শুরু করতে পারছেন না
অলরেডি প্রোগ্রামার হিসেবে কাজ করছেন এবং চাচ্ছেন AI ইঞ্জিনিয়ার হিসেবে ক্যারিয়ার স্টার্ট করতে
কোর্স থেকে কী কী শিখতে পারবেন?
Python প্রোগ্রামিং — AI এর জন্য যেটা সবচেয়ে জরুরি
প্রয়োজনীয় ম্যাথমেটিক্স ও স্ট্যাটিস্টিক্স — ব্যাকএন্ডে যা সব কিছুর বেস
NLP (Natural Language Processing) — ল্যাংগুয়েজ বোঝা ও আন্ডারস্ট্যান্ড করা
LLM (Large Language Models) — যেমন GPT-এর মতো মডেল কিভাবে কাজ করে
LangChain — LLM-কে কাজে লাগিয়ে প্রজেক্ট বানানো শিখবেন
Vector Database — AI মডেল ট্রেইনিংয়ের জন্য দরকারি ডেটা ম্যানেজমেন্ট
Speech Recognition — ভয়েস কমান্ড আর অডিও প্রসেসিং শেখা
Real-world Projects — পুরো কোর্সে ১২টা রিয়েল প্রজেক্ট বানাবেন নিজের হাতে!
কী কী প্রোজেক্ট করানো হবে কোর্সে?
House Price Predictor (Regression)
Diabetes Detection System (Classification)
Customer Segmentation Engine (Unsupervised)
MNIST Digit Classifier (Deep Learning)
Dog vs Cat Classifier (CNN)
Sales Predictor (Time-Series)
Sentiment Analyzer (NLP)
Resume Generator (LLM)
Business Assistant Chatbot (LangChain Tools)
Document QA Chatbot (LangChain + PDF)
Research Assistant (Multi-Agent)
Knowledge Base Chatbot (RAG)
Final Capstone: End-to-End AI Application with Deployment
কোর্স চলাকালীন প্রজেক্টসমুহ
রিকোয়ারমেন্টস
আগে থেকে পাইথন প্রোগ্রামিং এর উপর নলেজ থাকা লাগবে।
হেল্প
ব্যাচ সংক্রান্ত যেকোনো তথ্যের জন্যে কল করুন+88013038047939(সকাল ১০টা থেকে রাত ১০টা)
ক্যারিয়ার কাউন্সিলরের সাথে কথা বলুন